Introduction
Welcome to the fascinating world of seasonal stock algorithms and stock market predictions, where data becomes the compass guiding retail investors through the cyclical waves of the market. The concept of seasonality in stock market investments is not just a myth or an old trader’s tale; it’s a statistically significant trend that has captured the attention of both novice and seasoned investors alike. As we delve into the intricate dance of numbers and patterns, let’s unlock the secrets that seasons hold over the stock market’s heartbeat.
Understanding Seasonal Stock Market Trends
Seasonality is the market’s pulse, a rhythm set by the calendar, echoing through the corridors of finance with patterns that savvy investors seek to understand and harness. The stock market, much like nature, goes through its own seasons, with assets and sectors blossoming or withering away in predictable cycles. (Decoding Seasonal Stock Patterns) provides a deeper dive into how these temporal shifts influence market trends and trader behavior.
Machine Learning: The Future of Financial Forecasting
The advent of machine learning has revolutionized the art of financial forecasting, turning seasonal stock algorithms into a science of precision and foresight. By feeding historical data into the voracious algorithms of machine learning systems, we can unveil the subtle seasonal nuances that drive stock performance. From Random Forests to Deep Neural Networks, these technological maestros parse through the data, learning and adapting, to offer predictions that arm investors with the foresight once thought impossible.
Data-Driven Investment Strategies: A Game Changer
In the modern arena of algorithmic trading, data-driven investment strategies stand as the champions of informed decision-making. Retail investors now wield the power to sift through the noise and pinpoint seasonal patterns that spell opportunity and risk mitigation. By embracing advanced investment strategies, they can navigate the stock market with a map drawn from the very fabric of historical trends, machine learning insights, and financial forecasting prowess.
The benefits of this approach are manifold; from improved profitability to enhanced risk management, the data-driven methodology is reshaping how we perceive and engage with the ebb and flow of the markets. It’s a transformation that invites investors to not just ride the seasonal waves, but to predict them, to master them, and ultimately, to profit from them.
Join us on this journey as we explore the intricacies of seasonal stock algorithms and unveil the potential of machine learning in shaping the future of stock market predictions. Together, let’s crack the code and discover how to leverage the seasons in our investment strategies for a brighter, more prosperous trading tomorrow.
The Role of Machine Learning in Seasonal Predictions
In the dynamic world of stock markets, seasonal stock algorithms and machine learning are converging to create powerful investment strategies. As retail investors and financial institutions alike strive to gain an edge, machine learning emerges as a pivotal tool in dissecting and leveraging seasonal trends for stock market predictions.
Machine Learning and Stock Market Synergy
Machine learning, a subset of artificial intelligence, is revolutionizing the way we understand and predict market behaviors. It excels in sifting through vast amounts of historical stock data, financial news, and investor behavior patterns to unearth valuable insights. By employing sophisticated algorithms, machine learning models can detect subtle signals that may indicate upcoming seasonal shifts, enabling investors to anticipate market movements with greater precision.
But what exactly is machine learning’s role within the realm of seasonal stock algorithms? It’s simple: it provides the computational might to process complex datasets and identify the cyclical patterns that can often elude the human eye. This data-driven approach equips investors with forecasts that are continuously refined as new information is assimilated, ensuring that each prediction is more accurate than the last.
Harnessing Seasonal Patterns with AI
Investors looking to capitalize on seasonal stock trends can turn to advanced machine learning models, such as Random Forests and Deep Neural Networks. These models are adept at capturing the nuances of seasonal patterns that are influenced by a myriad of factors, including market cycles, holiday periods, and even weather changes.
To harness these seasonal patterns, investors must first gather and preprocess relevant data. This can include historical sales figures, inventory levels, and other external variables that bear on stock performance. With this data in hand, machine learning algorithms can then forecast seasonal demand with a high degree of accuracy, providing a solid foundation for algorithmic trading and investment strategies.
Building Blocks of Seasonal Forecasting Models
Developing a successful machine learning model for seasonal predictions hinges on several crucial components. The incorporation of lagged variables, rolling averages, and other statistical techniques can help account for the inherent seasonality in stock market data. Choosing the right algorithm is equally important, as it must align with the specific characteristics of the data being analyzed.
Dividing data into training and testing sets is a critical step in validating the model’s effectiveness. Investors must rigorously assess model performance and continuously refine hyperparameters to ensure the accuracy of predictions. By combining different models, an ensemble approach can further enhance prediction reliability and minimize potential biases.
Practical Applications for Retail Investors
Retail investors can leverage these machine learning insights to inform their investment strategies. By understanding the anomalies that machine learning can unearth in stock market seasonality, investors are better positioned to make strategic decisions that align with anticipated market movements.
For those curious about the intricacies of these anomalies and how machine learning can detect them, further insights can be found in our detailed analysis (Understanding Seasonal Stock Anomalies).
Conclusion
Machine learning is undeniably transforming the landscape of financial forecasting. Its ability to analyze and interpret complex seasonal data has opened new horizons for investment strategies. As retail investors and financial professionals increasingly adopt these data-driven approaches, the stock market’s seasonal rhythms will continue to be decoded with ever-growing sophistication. With machine learning at the helm, the ability to forecast and capitalize on seasonal trends is becoming more accessible, paving the way for a new era of algorithmic trading and informed investment decision-making.
Developing Algorithms for Seasonal Stock Forecasting
Investing in the stock market can often seem like a complex dance with time, where each step is dictated by an underlying rhythm of seasons. Seasonal stock algorithms are at the heart of decoding this rhythm, enabling investors to choreograph their moves with greater precision. This section delves into the nuanced process of creating algorithms designed for seasonal stock forecasting and the pivotal role they play in stock market predictions.
The Genesis of Seasonal Forecasting Models
The journey of developing a seasonal stock algorithm begins with historical data. By calculating historical averages, data scientists and financial analysts establish a foundational understanding of past market trends. This retrospective analysis sets the stage for machine learning techniques to enter the fray, bringing tools like Random Forests, Gradient Boosting, and Deep Neural Networks to the forefront. These algorithms are trained to not only recognize existing patterns but also to predict future market movements with a substantial degree of accuracy.
Collecting and preprocessing data is a cornerstone of this process. It involves meticulous gathering of historical information, such as sales data and inventory levels, and may also consider the influence of (external factors), like weather, that impact market seasonality. Data scientists then embark on cleaning this data, which includes outlier removal and missing value imputation, ensuring that the input to the forecasting models is as accurate and reliable as possible.
Crafting the Algorithmic Blueprint
Once the data is primed, the actual crafting of the algorithm begins. This involves the identification of seasonal patterns and determining the forecasting horizon—critical steps that distinguish seasonal stock algorithms from generic financial prediction models. Seasonal algorithms are specifically engineered to harness the cyclical nature of markets, differentiating them from broader financial models that might focus on a wider range of predictive factors.
Selecting the right model is a delicate balance; the complexity must be just enough to capture seasonality without succumbing to overfitting or underfitting. This is a key challenge in the development process, as is the need to model multi-seasonal patterns and to account for abrupt changes in market behavior.
Adapting to Market Ebbs and Flows
The true test of any seasonal stock algorithm lies in its adaptability. Financial markets are in constant flux, influenced by a myriad of factors both predictable and unforeseen. Seasonal algorithms must, therefore, include a mechanism for continuous learning and refinement, allowing them to evolve in response to new data. This agility is what makes them a powerful tool in the arsenal of modern investment strategies, particularly for retail investors and those engaged in algorithmic trading.
In the realm of financial forecasting, the implementation of these algorithms is not without its challenges. The intricacies of algorithmic trading coupled with the volatile nature of the stock market demand a robust framework that can withstand the test of time and the unpredictability of market forces.
Empowering Retail Investors through Algorithmic Insights
Finally, it is the retail investor who stands to gain the most from these advancements in seasonal stock forecasting. With access to sophisticated machine learning-driven insights, the individual investor can now make data-backed decisions that were once the domain of institutional traders. These algorithms level the playing field, providing retail investors with a glimpse into the cyclical undercurrents of the stock market and empowering them to build more resilient investment strategies.
In conclusion, the development of algorithms for seasonal stock forecasting is a meticulous and dynamic process, one that intertwines machine learning expertise with deep financial acumen. As we continue to refine these models, they will undoubtedly become a staple in the investor’s toolkit, enhancing the strategic approach to the ever-changing tapestry of the stock market.
Case Studies: Successes and Failures in Seasonal Prediction
Understanding the intricacies of seasonal stock algorithms and their outcomes in stock market predictions is pivotal for investors and financial analysts. Through examining case studies of both triumphs and slip-ups, we gain insights into the potential and limitations of harnessing machine learning in financial forecasting. Let’s delve into real-world instances that illuminate the path to more robust investment strategies, particularly for retail investors engaged in algorithmic trading.
Success Stories: Riding the Seasonal Waves
One of the prominent success stories in the use of seasonal stock algorithms is the well-documented January Effect. This phenomenon suggests that stocks, especially those of small-cap companies, tend to experience a surge in prices during January more than other months. Investors who capitalize on this trend by buying in December and selling after the January rally have historically reaped benefits. The seasonal stock algorithm that predicts this trend exemplifies how understanding market cycles can lead to successful investment strategies.
Another success case involves the retail sector, where algorithms anticipate increased consumer spending during holiday seasons. By adjusting their portfolio to include retail stocks ahead of the festive rush, savvy investors have been able to secure substantial gains. These algorithms take into account not just historical sales data, but also consumer sentiment indicators, providing a more comprehensive prediction model.
Learning from Failures: Cautionary Tales
Despite the allure of algorithmic trading and seasonal stock predictions, we must also consider the setbacks. A noteworthy failure occurred when seasonal algorithms did not account for an economic recession, resulting in predictions that were wildly off the mark. This has taught investors the importance of incorporating broader economic indicators into their models, rather than relying solely on seasonal patterns.
In another instance, the food and beverage industry suffered from inaccurate seasonal inventory forecasting. This led to excessive waste and loss of revenue, highlighting the risks associated with over-reliance on historical data without considering potential market shifts. Such failures underscore the need for dynamic models that can adapt to unusual market conditions and incorporate real-time data.
Refining the Approach: Lessons for Future Models
Every success and failure in seasonal stock predictions contributes to the ongoing evolution of forecasting models. For instance, the complexities of the financial market demand that models avoid overfitting to past data, which can render them ineffective in predicting future trends. Machine learning offers a solution by allowing algorithms to learn and adjust to new data, making them more resilient to the unpredictable nature of the stock market.
Retail investors can benefit from these advancements in financial forecasting. By utilizing machine learning algorithms that have been refined through trial and error, they can make more informed decisions based on sophisticated investment strategies.
The case studies also emphasize the necessity of what-if scenarios in algorithm development. These scenarios help model the impact of unforeseen events, such as geopolitical shifts or global health crises, on stock market predictions. By preparing for uncertainties and integrating external data APIs, future prediction models can offer more reliable guidance to investors.
Moving Forward: Algorithmic Trading for the Retail Investor
The insights gained from these case studies are invaluable for retail investors who are increasingly turning to algorithmic trading as a means to navigate the stock market. By learning from the successes and failures of seasonal stock predictions, they can adopt investment strategies that are data-driven and equipped to handle the complexities of market seasonality.
As machine learning continues to revolutionize financial forecasting, investors are poised to benefit from algorithms that are not only predictive but also prescriptive, offering actionable insights that go beyond traditional analytics. With each case study, the financial industry moves closer to unlocking the full potential of seasonal stock algorithms, empowering investors with the tools they need for strategic decision-making in the ever-evolving marketplace.
Practical Applications for Retail Investors
Retail investors, armed with the right knowledge and tools, can utilize seasonal stock algorithms to enhance their investment strategies. Understanding and leveraging stock market predictions can provide a competitive edge, allowing for the strategic timing of trades and portfolio adjustments. Here’s how you can apply machine learning and algorithmic trading to your financial forecasting endeavors.
Harnessing Seasonal Algorithms for Portfolio Growth
Seasonal stock algorithms offer a data-driven approach to identifying potential opportunities within the market. By analyzing historical patterns, these algorithms can highlight times when certain stocks or sectors have consistently performed well or poorly. Retail investors can use this information to adjust their holdings accordingly—buying, selling, or reallocating assets to optimize returns.
Integrating Machine Learning into Decision-Making
Machine learning has revolutionized financial forecasting by providing the ability to digest vast amounts of data and uncover complex patterns. For retail investors, embracing machine learning tools can lead to more nuanced insights into seasonal trends. Platforms like Google Cloud, Azure Machine Learning, and AWS Machine Learning ((Google Cloud)[https://cloud.google.com/], (Azure Machine Learning)[https://azure.microsoft.com/en-us/services/machine-learning/], and (AWS Machine Learning)[https://aws.amazon.com/machine-learning/]) offer robust solutions for analyzing stock market data and developing predictive models.
Crafting Diversified Seasonal Strategies
While seasonal stock predictions can be compelling, they should not be the sole basis for trading decisions. Retail investors should aim to build a diversified investment strategy that incorporates seasonality as one of many factors. This approach mitigates risks and ensures that one’s portfolio isn’t overly exposed to the potential shortcomings of seasonal forecasts, such as data anomalies or unexpected market shifts.
Navigating Tools for Seasonal Predictions
A variety of tools are available to retail investors seeking to access seasonal prediction algorithms. From historical averages for rudimentary pattern recognition to advanced deep learning models like RNNs and LSTMs, these technologies can aid in capturing intricate seasonal patterns. Retail investors can explore the use of specific algorithms, such as Facebook’s Prophet, or dive into complex machine learning models to develop their own bespoke forecasting systems.
Risk Management in Seasonal Trading
When it comes to algorithmic trading based on financial forecasting, risk management is paramount. Retail investors must be vigilant, ensuring that their strategies account for the dynamic nature of the stock market. They should also be cautious of over-reliance on past patterns, considering the likelihood that market conditions can and do change, rendering some seasonal trends obsolete.
Strategic Implementation for the Individual Investor
For the individual investor, the strategic implementation of seasonal stock predictions requires both a discerning eye and a balanced approach. Regularly reviewing and adjusting one’s investment strategies in light of new data and market developments is key. This proactive stance allows retail investors to stay ahead of the curve, capitalizing on the predictive power of seasonal stock algorithms while navigating the ever-changing stock market landscape.
By carefully considering these practical applications, retail investors can effectively incorporate seasonal stock algorithms into their investment strategies. With the right mix of machine learning insights, diversified strategies, and robust risk management, you can unlock the potential of seasonal trends to inform and enhance your financial decision-making.
FAQ: Navigating Seasonal Stock Algorithms and Predictions
Reliable Sources for Seasonal Predictions
Question: What is the most reliable source for seasonal stock predictions?
Answer: For investors looking to tap into the power of seasonal stock algorithms, it’s essential to draw on the strength of machine learning and historical data. Tools that combine Random Forests, Gradient Boosting, and Deep Neural Networks are at the forefront, offering a deep dive into past trends to forecast future market behaviors. Additionally, the utilization of sophisticated forecasting tools like Prophet ensures that even the most nuanced seasonal patterns don’t go unnoticed. For a more contextual insight, engaging with industry players to interpret consumer behaviors during diverse seasons can further sharpen the accuracy of stock market predictions.
Retraining Seasonal Algorithms
Question: How often should algorithms be updated or retrained?
Answer: Seasonal stock algorithms are not set-and-forget; they thrive on adaptation. The dynamic nature of the stock market dictates the retraining frequency of these models. Quarterly or annual updates are common practices to keep the algorithms in sync with the market’s pulse. However, the true compass for retraining should be the data itself—monitor for drift, analyze the shifts, and let the market’s evolution guide the retraining schedule. This ensures that the investment strategies rooted in these predictions remain relevant and effective.
Seasonal Predictions & Investment Strategies
Question: Can seasonal stock predictions be integrated with other investment strategies?
Answer: Absolutely. Seasonal stock predictions are a strategic ally to a diversified investment portfolio. They can be woven into a tapestry of investment strategies, complementing both fundamental and technical analyses. By considering the cyclical nature of the markets, investors can leverage calendar-based strategies, sector rotations, and event-based trades to enhance their overall investment approach. The key is to blend seasonality with a rigorous understanding of market fundamentals and to apply steadfast risk management practices to capitalize on these recurring patterns.
Legal Aspects of Algorithmic Predictions
Question: Are there any legal considerations to be aware of when using algorithmic predictions?
Answer: The legal landscape surrounding algorithmic trading and financial forecasting is intricate. It’s imperative for retail investors and algorithmic trading professionals to ensure that their strategies comply with the ethical standards and regulations governing the financial markets. This means prioritizing fairness and transparency in algorithm development and being vigilant about the potential for bias and discrimination. Operators must continuously evaluate the socio-economic impact of their algorithms, ensuring adherence to anti-discrimination laws and taking proactive steps to counteract any form of bias.
Incorporating seasonal stock algorithms into your investment strategies can offer a significant edge in the stock market. For retail investors interested in algorithmic trading and machine learning, it’s crucial to stay informed and agile. By understanding the intricacies of financial forecasting and the legalities involved, you can harness these tools to make more data-driven decisions and potentially grow your portfolio with the changing seasons.
Conclusion
As we stand on the cusp of a new era in stock market predictions, it’s clear that seasonal stock algorithms have carved out a significant niche in the realm of financial forecasting. Armed with data-driven insights and the computational power of machine learning, these algorithms offer retail investors a valuable lens through which to view the cyclical ebbs and flows of the markets.
Embracing the Seasonal Edge
In the quest for portfolio growth, investors who leverage algorithmic trading stand to benefit from the predictive strength of seasonal patterns. While not a crystal ball, these tools provide a strategic advantage by highlighting potential opportunities that align with historical trends. Investment strategies that incorporate machine learning not only sharpen the investor’s competitive edge but also contribute to a more nuanced understanding of market dynamics.
The Algorithmic Symphony
The synergy between machine learning and seasonal stock algorithms is producing a harmonious blend of technology and traditional market wisdom. As retail investors gain access to these sophisticated tools, the democratization of algorithmic trading is empowering even the most casual market participants to make informed decisions backed by robust data analysis.
Investing with Foresight
In conclusion, while the effectiveness of seasonal algorithms is subject to market volatility and unforeseen events, they remain an indispensable part of a diversified investment approach. Investors who adapt to the rhythm of the markets, using algorithmic insights as a guide, can navigate the complexities of financial forecasting with greater confidence.
Looking ahead, the continued refinement of these algorithms and the integration of broader market factors promise to enrich the landscape of stock market predictions. For retail investors, it’s an exciting time to harness the power of seasonal stock algorithms, crafting investment strategies that are both resilient and responsive to the times.
As we continue to witness the evolution of this field, one thing remains certain: The intelligent application of seasonal algorithms will continue to shape the future of investment decision-making, offering a seasonal edge to those ready to embrace the potential within the patterns.
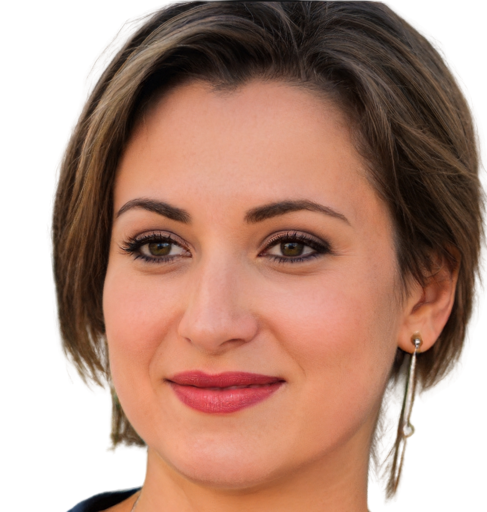
“Make the invisible visible. My goal is to shine a light on the subtle seasonal signals in the stock market, providing investors with the insight needed to make informed decisions. By breaking down the complexities of seasonality, I strive to empower our audience with knowledge and foresight, turning data into action.”